The algorithm, dubbed "RegRank," was unveiled late last week in a
new paper authored by the Commodity Futures Trading Commission's
former Chief Economist Andrei Kirilenko, University of Maryland
professor Shawn Mankad, and University of Michigan professor George
Michailidis.
The algorithms and statistical tests at the heart of the paper are
fairly standard. But what the researchers say is unique is how they
use these empirical tools to measure "regulatory sentiment" and
"test the impact" of the public comment process on rulemaking.
Such a tool could come in handy for regulators such as the CFTC and
Securities and Exchange Commission, both of whom have faced legal
challenges to rules on the grounds that regulators failed to
properly incorporate feedback, as required by law.
"The regulators need to be accountable to the public," Kirilenko
said of the rulemaking process.
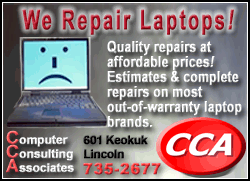
It could also be helpful for the industry and the public, as a way
to ensure that regulators heed feedback about flaws in proposed
reforms. The paper can be found at
http://papers.ssrn.com/sol3/
papers.cfm?abstract_id=2377826.
Kirilenko, now a professor at the Massachusetts Institute of
Technology's business school, told Reuters in an interview the idea
stems from his time as CFTC chief economist.
In that role, Kirilenko was in charge of reviewing cost-benefit
analyses for new rules for over-the-counter derivatives and helping
to ensure public comments — drafted by lawyers, lobbyists and
industry insiders — were weighed.
During that process, he realized all the legal jargon often used in
the comments could actually be boiled down to clusters of "basic
words," he said.
"I thought this is an interesting operation," he said. "Let's see if
we can actually uncover this hidden structure using a computer
algorithm."
The technology is an automated "machine-learning" method that works
by mining regulatory text, which can span hundreds of pages. The
algo searches for certain clusters of key words that are deemed
"pro-regulation" or "anti-regulation."
To measure whether words are positive or negative, the researchers
combined two publicly available dictionaries created by computer
scientists that label words with a positive or negative tone.
Positive words could include, for instance, "bullish," "affordable"
or "advantageous," while negative words include "exacerbate" or
"corrupt."
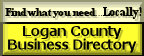
[to top of second column] |
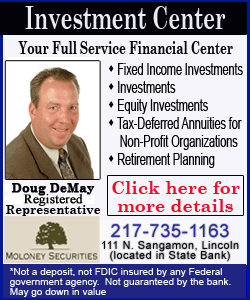
The algo is then able to keep score of how often such pro or
anti-regulatory words appear, allowing researchers to compare how a
proposed rule evolves into a final one and whether the public's
comments were heeded.
The topic has gained more attention as regulators have drafted
hundreds of new rules required by the 2010 Dodd-Frank Wall Street
reform law.
The Administrative Procedures Act (APA) requires many federal
agencies to carefully weigh public feedback, provide notice and
comment, and afford due process in general before rules are
finalized.
The CFTC is currently facing a legal challenge by three Wall Street
trade groups over its policy governing how U.S. swaps rules should
apply overseas.
The groups allege the agency violated the APA, in part by failing to
seek proper public comments and weigh costs and benefits.
As a testing ground for the "RegRank" algo, the three researchers
said in their January 11 paper that they examined 104 proposed and
67 final rules at the CFTC, as well as 60,000 public comments
submitted between January 2010 and September 2013, as the agency was
busy drafting new rules for the over-the-counter derivatives market
required by the Dodd-Frank law.
The researchers determined that "the government agency does adjust
the final rule in response to public comments."
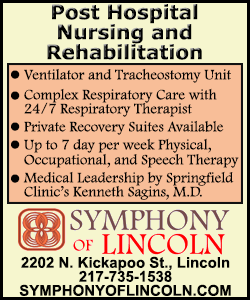
The paper still must be peer-reviewed, and the researchers plan to
present it at conferences.
"The results show that the government listens, but more research
would be needed for deeper insights," said Mankad.
(Editing by Bernadette Baum)
[© 2014 Thomson Reuters. All rights
reserved.] Copyright 2014 Reuters. All rights reserved. This material may not be published,
broadcast, rewritten or redistributed. |