The Institute of Medicine (now the National Academy of Medicine)
says clinical practice guidelines should be based on a systematic
review of the evidence, lead author Dr. Paul Shekelle from RAND
Corporation in Santa Monica, California, told Reuters Health by
email.
“We know that clinical practice guidelines go out of date over time,
as new evidence accumulates. An impediment to the regular updating
of clinical practice guidelines is the time and resources needed to
update the systematic review,” he said.
Typically, researchers and their assistants perform computer
searches to identify anywhere from a few to thousands of new
research studies, then they determine which ones are relevant and
assemble the information into updated guidelines and
recommendations.
Shekelle and colleagues thought machines could do more of the job
and do it faster, so they compared machine-learning methods with the
standard search methods for identifying new information.
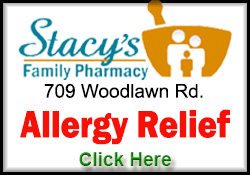
They tested the idea on three health conditions: gout, low bone
density and osteoarthritis of the knee. The smart search program
“learned” which key terms to look for by analyzing words from
studies that were included in prior reviews on each topic.
In all three cases, computers - provided only with the titles and
summaries of articles included in previous reviews - reduced the
number of articles researchers had to screen further by 67 to 83
percent, according to the results in Annals of Internal Medicine.
The machine-learning method missed only two articles that humans
would have identified, for an overall accuracy of 96 percent. And
neither of these articles would have changed the ultimate evidence
reviews, Shekelle’s team concludes.
“Machine learning methods are very promising as a way to reduce the
amount of time and effort for the literature search, which in turn
should make it easier to update the systematic review, which in turn
can facilitate keeping clinical practice guidelines up to date,”
Shekelle said.
[to top of second column] |
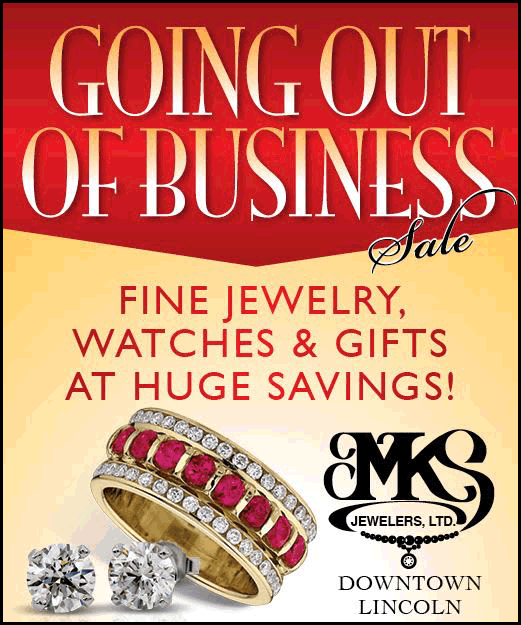
The approach would “shorten the time from completion of research
studies to adoption of effective treatments in clinical practice,”
said Dr. Alfonso Iorio from McMaster University in Hamilton,
Ontario, Canada, who coauthored an editorial accompanying the
report.
“Also, it will allow more efficient update of doctors about what
works and what does not, saving lives and dollars,” he said by
email.
Iorio thinks this method would have the greatest impact in the
fields of cardiology, diabetes, respiratory disease and cancer. “But
any field would benefit,” he said.
“In the near future, artificial intelligence will also be used to
match to individual need with the best available health care
intervention - one necessary step to get this is proper
classification on existing and newly generated knowledge,” Iorio
said.
“The critical step is training properly the computer systems - we
need to ensure research dollars are provided to ensure this training
is done by serious and independent researchers and controlled by
public institutions.”
SOURCE: http://bit.ly/2rslYlR and http://bit.ly/2rhBLA0 Annals of
Internal Medicine, online June 13, 2017.
[© 2017 Thomson Reuters. All rights
reserved.] Copyright 2017 Reuters. All rights reserved. This material may not be published,
broadcast, rewritten or redistributed.
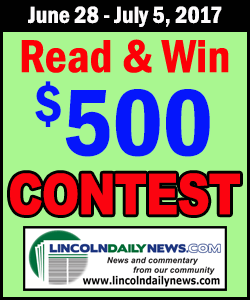 |