That’s why economists, agricultural researchers, government
agencies, and private companies are working to improve the
accuracy of these predictions.
The U.S. Department of Agriculture’s monthly World Agricultural
Supply and Demand Estimates (WASDE) report forecasts
end-of-season yield based on real-time farmer surveys and
analysis from the USDA National Agricultural Statistics Service
(NASS), and it’s considered by many to be the gold standard for
yield predictions. But researchers at the University of Illinois
have developed a new method that outperforms the USDA’s WASDE
estimations, in a scientifically rigorous and reproducible way.
“Using seasonal forecasts and satellite data, we developed a
very advanced yield prediction system for both the national and
county levels. Our research demonstrates that we can do better
than the USDA’s real-time estimation,” says Kaiyu Guan,
principal investigator on the Geophysical Research Letters
study, and assistant professor in the Department of Natural
Resources and Environmental Sciences (NRES) at U of I and Blue
Waters professor at the National Center for Supercomputing
Applications (NCSA).
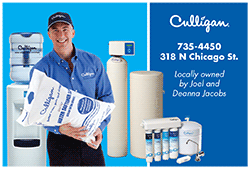
Guan and his colleagues are not the first to use satellite data
to try to predict crop yield, but their combined use of seasonal
climate prediction, along with crop growth information from
satellite imagery, is unique.
The study evaluated end-of-season accuracy of individual and
combined data sources as compared with the national maize yield
forecast in the monthly USDA WASDE reports.
“Compared with using historical climate information for the
unknown future, which is what most previous research is based
on, using seasonal climate prediction from the NOAA’s National
Centers for Environmental Prediction gave better forecasting
performance, especially in reducing the uncertainties,” says Bin
Peng, the lead author of this study and postdoctoral research
associate in NRES and NCSA.
[to top of second column] |
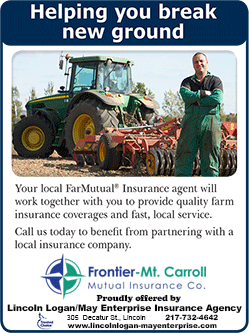
Guan adds, “But if we only use seasonal climate
prediction data – temperature, rainfall, and vapor pressure deficit
– our predictions were no better than the USDA’s. It was only when
we added the satellite data that we started to see the improvement.
That’s a clear indication that satellite data is extremely useful in
this case.”
The new approach allows more accurate end-of-season predictions to
be made earlier in the season. At the end of the growing season,
when the corn harvest is complete, it is possible to look back and
evaluate the accuracy of each previous month’s prediction. Between
2010 and 2016, for example, the WASDE report for June was off, on
average, by 17.66 bushels per acre. For the same time frame, Guan
and Peng’s system was only off by 12.75 bushels per acre. In August,
WASDE was off by an average of 5.63 bushels per acre, whereas Guan
and Peng’s system got the number down to 4.37.
“Improving our ability to predict crop yield is really important for
many applications. Farmers want to know this information because
it’s directly related to the price they can expect. Economic
forecasting and commodity market prices for corn and soybean hinge
on this information. Also logistics: Grain companies need to find
out which places are producing grain and how much. Do they have the
capacity to collect and process it? Crop insurance depends on this
information, too. It’s a problem that has lots of practical
meaning,” Guan says.
“The new system is implemented on Blue Waters, one of the most
advanced supercomputers in the U.S.,” says Peng. “We need a
high-performance computing facility like Blue Waters to process the
huge amount of seasonal climate prediction and satellite data.”
The article, “Benefits of seasonal climate prediction and satellite
data for forecasting U.S. maize yield,” is published in Geophysical
Research Letters [DOI: 10.1029/2018GL079291]. Additional co-authors
include Ming Pan of Princeton University and Yan Li of NRES at U of
I. The research was supported by NASA, USDA NIFA, and NCSA, which
has additional support from the NSF and the state of Illinois for
its Blue Waters supercomputer.
[ACES News] |