In a novel approach to the problem, the authors turned to
crowdsourcing to help them develop a computer algorithm that would
take over some of the duties of an experienced radiation oncologist.
"Lung cancer is a major global health problem," said the study's
lead author, Dr. Raymond Mak, an associate professor of radiation
oncology at Harvard Medical School, the Dana Farber Institute and
Brigham and Women's Hospital in Boston. "It's the number one cancer
killer in the world. Close to a million patients will need radiation
therapy at some point."
Currently, radiation oncologists determine where to send the beams
of radiation by drawing the outlines of the tumor and surrounding
organs. The first step is to do a CT scan of the patient's tumor and
surrounding tissues. The scan will produce multiple cross-sectional
images, or "slices," which together effectively yield a 3D image.
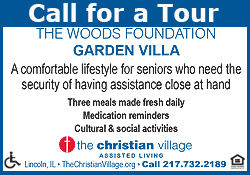
"You take your medical imaging - there can be 100 images that are
slices through the tumor - and hand-draw on each slice where the
tumor is," Mak explained. "Then you draw in where the organs are.
And then you determine how to aim the radiation."
That prep can take hours, Mak said. "It's very time consuming," he
added. "And there's lots of variation even between experts. When you
miss and the radiation doesn't go to the right place it can directly
impact patient care."
When you look at the issue from a global perspective, there's also
the problem of lack of access, Mak said. Many places do not have
enough specialists to draw the tumors accurately.
To determine whether artificial intelligence could fill the void,
Mak and his colleagues set up a contest on a website called
Topcoder.com that hosts challenges for a community of more than a
million programmers around the world, who compete for prizes by
solving computational problems.
In this case, the researchers had $50,000 in prize money to offer
coders who could come up with "new AI techniques that could train
machines to replicate an expert clinician's ability to target a
tumor," Mak said.
A total of 564 contestants from 62 countries registered for the
challenge, which was to design a program that would allow a computer
to look at multiple expert-drawn tumors and thereby learn to draw
itself.
[to top of second column] |
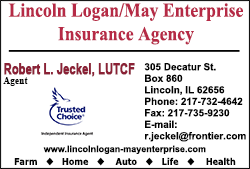
In the end, 10 independent winning algorithms, filed by 9
participants, were chosen. Then the top five algorithms were
combined into one "ensemble" program, which did fairly well when put
up against the work of actual radiation oncologists.
"We compared the performance of the algorithms, that is, compared
algorithm-generated (drawings) versus the human expert to generate a
performance score on each case, and then benchmarked against the
variation seen between multiple human experts against the study's
expert, and also the intra-observer variation, that is the same
expert doing the same task twice," Mak said. "The ensemble of the
best algorithms had overlap scores in 75 percent of the cases that
matched intra-observer score."
The new approach may help fill the global shortage of radiation
oncologists, said Dr. Sushil Beriwal, a professor of radiation
oncology and deputy director of radiation services at the Hillman
Cancer Center at the University of Pittsburgh Medical Center in
Pennsylvania.
"One of the biggest challenges to targeting tumors is the lack of
manpower," said Beriwal, who was not involved in the new research.
"The eventual goal is to come out with a product that can be used to
help where an expert is not available and to use as a second check
(of the radiation oncologist's own work)."
In the final iteration of the project, "they were very close to the
variation you would expect between physicians," Beriwal said.
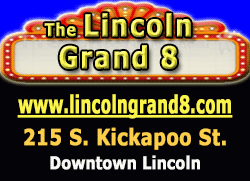
Dr. Nicholas Sanfilippo welcomed the new research. "It's an exciting
new technology," said Sanfilippo, a radiation oncologist at NewYork-Presbyterian/Weill
Cornell Medical Center in New York City, who also wasn't involved in
the study. "As clinicians we should look to making it complement the
care of the physician, especially in underserved areas."
SOURCE: https://bit.ly/2VNQrWF and https://bit.ly/2UrxRSy JAMA
Oncology, online April 18, 2019.
[© 2019 Thomson Reuters. All rights
reserved.] Copyright 2019 Reuters. All rights reserved. This material may not be published,
broadcast, rewritten or redistributed.
Thompson Reuters is solely responsible for this content. |