The research, published in Remote Sensing of Environment,
combines field measurements, a unique in-field camera network,
and high-resolution, high-frequency satellite data, providing
highly accurate productivity estimates for crops across Illinois
and beyond.
“Our ultimate goal is to provide useful information to farmers,
especially at the field level or sub-field level. Previously,
most available satellite data had coarse spatial and/or temporal
resolution, but here we take advantage of new satellite products
to estimate leaf area index (LAI), a proxy for crop productivity
and grain yield. And we know the satellite estimates are
accurate because our ground measurements agree,” says Hyungsuk
Kimm, a doctoral student in the Department of Natural Resources
and Environmental Sciences (NRES) at U of I and lead author on
the study.
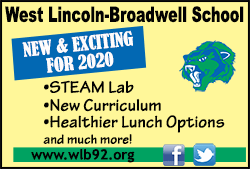
Kimm and his colleagues used surface reflectance data, which
measures light bouncing off the Earth, from two kinds of
satellites to estimate LAI in agricultural fields. Both
satellite datasets represent major improvements over older
satellite technologies; they can “see” the Earth at a fine scale
(3-meter or 30-meter resolution) and both return to the same
spot above the planet on a daily basis. Since the satellites
don’t capture LAI directly, the research team developed two
mathematical algorithms to convert surface reflectance into LAI.
While developing the algorithms to estimate LAI, Kimm worked
with Illinois farmers to set up cameras in 36 corn fields across
the state, providing continuous ground-level monitoring. The
images from the cameras provided detailed ground information to
refine the satellite-derived estimates of LAI.
The true test of the satellite estimates came from LAI data Kimm
measured directly in the corn fields. Twice weekly during the
2017 growing season, he visited the fields with a specialized
instrument and measured corn leaf area by hand.
In the end, the satellite LAI estimates from the two algorithms
strongly agreed with Kimm’s “ground-truth” data from the fields.
This result means the algorithms delivered highly accurate,
reliable LAI information from space, and can be used to estimate
LAI in fields anywhere in the world in real time.
“We are the first to develop scalable,
high-temporal, high-resolution LAI data for farmers to use.
These methods have been fully validated using an unprecedented
camera network for farmland,” says Kaiyu Guan, assistant
professor in the Department of NRES and Blue Waters professor at
the National Center for Supercomputing Applications. He is also
principal investigator on the study.
[to top of second column] |
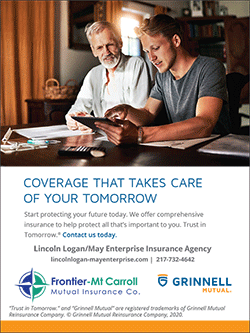
Having real-time LAI data could be instrumental for
responsive management. For example, the satellite method could
detect underperforming fields or segments of fields that could be
corrected with targeted management practices such as nutrient
management, pesticide application, or other strategies. Guan plans
to make real-time data available to farmers in the near future.
“The new LAI technology developed by Dr. Guan’s research team is an
exciting advancement with potential to help farmers identify and
respond to in-field problems faster and more effectively than ever
before,” says Laura Gentry, director of water quality research for
the Illinois Corn Growers Association.
“More accurate measurements of LAI can help us to be more efficient,
timely, and make decisions that will ultimately make us more
profitable. The last few years have been especially difficult for
farmers. We need technologies that help us allocate our limited
time, money, and labor most wisely. Illinois Corn Growers
Association is glad to partner with Dr. Guan’s team, and our farmer
members were happy to assist the researchers with access to their
crops in validating the team’s work. We’re proud of the advancement
this new technology represents and are excited to see how the Guan
research team will use it to bring value directly to Illinois
farmers,” Gentry adds.
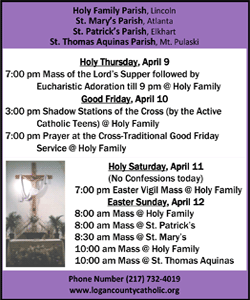
The article, “Deriving high-spatiotemporal-resolution leaf area
index for agroecosystems in the U.S. Corn Belt using Planet Labs
CubeSat and STAIR fusion data,” is published in Remote Sensing for
Environment [DOI: 10.1016/j.rse.2019.111615]. Co-authors include
Hyungsuk Kimm, Kaiyu Guan, Chongya Jiang, Bin Peng, Laura Gentry,
Scott Wilkin, Sibo Wang, Yaping Cai, Carl Bernacchi, Jian Peng, and
Yunan Luo. The work was supported by NASA programs, including NASA
New Investigator, NASA Carbon Monitoring System, and NASA Harvest
Program.
The Department of Natural Resources and Environmental Sciences is in
the College of Agricultural, Consumer and Environmental Sciences at
the University of Illinois.
[Sources: Hyungsuk Kimm,Kaiyu Guan,
Laura Gentry
News writer: Lauren Quinn] |