The idea is, always, to optimize corn yield for maximum profit.
Avoiding over-application is part of that calculation, but it's
also key to minimizing nitrogen pollution. So, which tool is
better?
"The difference between tools is small and difficult to see in
only a few trials. In consequence, we needed a dataset that
allowed us to compare performance in the long term. That is
where crop modeling came into play, allowing us to explore
millions of soil and weather combinations," says German Mandrini,
Ph.D. graduate from the Department of Crop Sciences at U of I,
and first author of the new study in Agricultural Systems.
Mandrini used a crop model based on thousands of fields across
Illinois to test static and dynamic nitrogen recommendation
tools.
"This is an interdisciplinary study, only attainable thanks to
the contributions of experts in crop modeling, environmental
sciences, agricultural economics, and crop sciences from several
institutions," says Mandrini. "The broad dataset not only
allowed us to compare the performance of the tools but also to
understand in what conditions the differences happened."
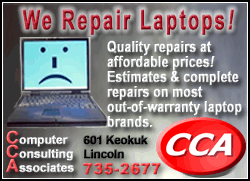
For the management scenarios explored, the researchers found
that complex dynamic tools did not consistently increase profits
over simpler static tools.
"Around half the time, dynamic tools under-predicted the amount
of nitrogen a farmer needs, leading to yield penalties. Those
yield penalties were usually high and not compensated by the
situations in which the dynamic tool predicts nitrogen with
higher accuracy,” says Nicolas Martin, corresponding author of
the study and assistant professor in the Department of Crop
Sciences. “For years, we haven't seen a clear winner among
nitrogen-prediction tools, and our results explain why."
Martin specializes in big-data approaches to agricultural
challenges, so the result came as a bit of a surprise. He
explains that the results are important for setting clear goals
in future research, acknowledging that higher complexity does
not always mean better results.
"Dynamic tools require bookkeeping and data input
from busy farmers, partially explaining the low adoption of
current products in the marketplace. From the farmer's point of
view, I imagine that newer tools based on precise information
represent extra work. And then it's not always clear how much
benefit they get one season to the other," Martin says. "I could
see if they ended up with a high yield penalty in one season,
farmers might not want to try it again."
[to top of second column] |
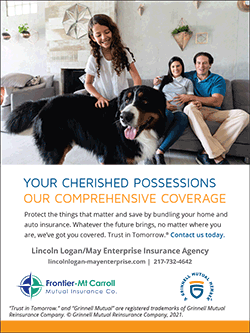
The researchers also found some good news from an
environmental standpoint: Both tools have the potential to reduce
nitrogen leaching by about 15% compared with current practices.
"The higher accuracy of dynamic tools leads to reduced leaching, but
static tools could achieve the same result by recommending the low
end of the MRTN range,” Mandrini says. “This reduction can be
attained in a simple way and at almost no cost for the farmers, just
by lowering current recommendations.”
Do these results mean complex dynamic nitrogen tools are doomed to
obscurity? The researchers don't think so. Instead, they say, the
findings are an opportunity to refine these tools and redefine their
goals. Since higher accuracy does not consistently improve profits
and reduce nitrogen leaching, developers of nitrogen recommendation
tools should be clear about whether they're prioritizing economic or
environmental outcomes.
“Our results highlight the need to develop additional strategies,
including education and policy, to account for environmental
benefits and provide clear incentives for farmers to adopt these
tools and increase the eco-efficiency of agriculture,” Martin says.
The article, "Understanding differences between static and dynamic
nitrogen tools using simulation modeling," is published in
Agricultural Systems [DOI: 10.1016/j.agsy.2021.103275]. Authors
include German Mandrini, Cameron Pittelkow, Sotirios Archontoulis,
Taro Mieno, and Nicolas Martin. This study was conducted thanks to
the support of NIFA\Multistate Hatch Grant: Enhancing nitrogen
utilization in corn-based cropping systems to increase yield,
improve profitability and minimize environmental impacts
[ILLU-802-965].
The Department of Crop Sciences is in the College of Agricultural,
Consumer and Environmental Sciences at the University of Illinois at
Urbana Champaign.
[Source: Nicolas Martin
News writer: Lauren Quinn]
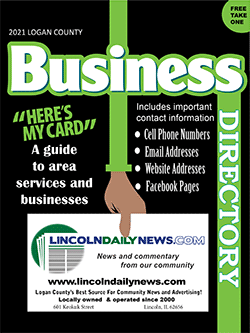 |