AI is explaining itself to humans. And it's paying off
Send a link to a friend
[April 06, 2022]
By Paresh Dave
OAKLAND, Calif. (Reuters) - Microsoft Corp's LinkedIn boosted
subscription revenue by 8% after arming its sales team with artificial
intelligence software that not only predicts clients at risk of
canceling, but also explains how it arrived at its conclusion.
The system, introduced last July and to be described in a LinkedIn blog
post on Wednesday, marks a breakthrough in getting AI to "show its work"
in a helpful way.
While AI scientists have no problem designing systems that make accurate
predictions on all sorts of business outcomes, they are discovering that
to make those tools more effective for human operators, the AI may need
to explain itself through another algorithm.
The emerging field of “Explainable AI,” or XAI, has spurred big
investment in Silicon Valley as startups and cloud giants compete to
make opaque software more understandable and has stoked discussion in
Washington and Brussels where regulators want to ensure automated
decision-making is done fairly and transparently.
AI technology can perpetuate societal biases like those around race,
gender and culture. Some AI scientists view explanations as a crucial
part of mitigating those problematic outcomes.
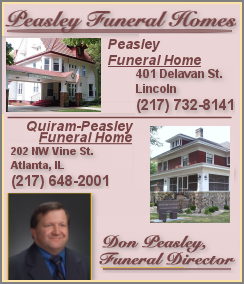
U.S. consumer protection regulators including the Federal Trade
Commission have warned over the last two years that AI that is not
explainable could be investigated. The EU next year could pass the
Artificial Intelligence Act, a set of comprehensive requirements
including that users be able to interpret automated predictions.
Proponents of explainable AI say it has helped increase the
effectiveness of AI’s application in fields such as healthcare and
sales. Google Cloud sells explainable AI services that, for instance,
tell clients trying to sharpen their systems which pixels and soon which
training examples mattered most in predicting the subject of a photo.
But critics say the explanations of why AI predicted what it did are too
unreliable because the AI technology to interpret the machines is not
good enough.
LinkedIn and others developing explainable AI acknowledge that each step
in the process - analyzing predictions, generating explanations,
confirming their accuracy and making them actionable for users - still
has room for improvement.
But after two years of trial and error in a relatively low-stakes
application, LinkedIn says its technology has yielded practical value.
Its proof is the 8% increase in renewal bookings during the current
fiscal year above normally expected growth. LinkedIn declined to specify
the benefit in dollars, but described it as sizeable.
Before, LinkedIn salespeople relied on their own intuition and some
spotty automated alerts about clients' adoption of services.
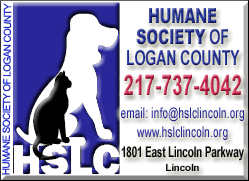
Now, the AI quickly handles research and analysis. Dubbed CrystalCandle
by LinkedIn, it calls out unnoticed trends and its reasoning helps
salespeople hone their tactics to keep at-risk customers on board and
pitch others on upgrades.
LinkedIn says explanation-based recommendations have expanded to more
than 5,000 of its sales employees spanning recruiting, advertising,
marketing and education offerings.
"It has helped experienced salespeople by arming them with specific
insights to navigate conversations with prospects. It’s also helped new
salespeople dive in right away," said Parvez Ahammad, LinkedIn's
director of machine learning and head of data science applied research.
[to top of second column]
|
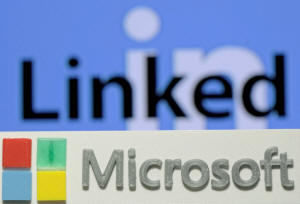
A 3D-printed logo of Microsoft is seen in front of a displayed
LinkedIn logo in this illustration taken June 13, 2016. REUTERS/Dado
Ruvic
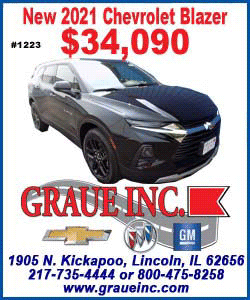 TO EXPLAIN OR NOT TO EXPLAIN?
In 2020, LinkedIn had first provided predictions without
explanations. A score with about 80% accuracy indicates the
likelihood a client soon due for renewal will upgrade, hold steady
or cancel.
Salespeople were not fully won over. The team selling LinkedIn's
Talent Solutions recruiting and hiring software were unclear on how
to adapt their strategy, especially when the odds of a client not
renewing were no better than a coin toss.
Last July, they started seeing a short, auto-generated paragraph
that highlights the factors influencing the score.
For instance, the AI decided a customer was likely to upgrade
because it grew by 240 workers over the past year and candidates had
become 146% more responsive in the last month.
In addition, an index that measures a client's overall success with
LinkedIn recruiting tools surged 25% in the last three months.
Lekha Doshi, LinkedIn's vice president of global operations, said
that based on the explanations sales representatives now direct
clients to training, support and services that improve their
experience and keep them spending.
But some AI experts question whether explanations are necessary.
They could even do harm, engendering a false sense of security in AI
or prompting design sacrifices that make predictions less accurate,
researchers say.
Fei-Fei Li, co-director of Stanford University's Institute for
Human-Centered Artificial Intelligence, said people use products
such as Tylenol and Google Maps whose inner workings are not neatly
understood. In such cases, rigorous testing and monitoring have
dispelled most doubts about their efficacy.
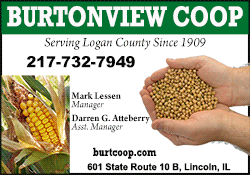
Similarly, AI systems overall could be deemed fair even if
individual decisions are inscrutable, said Daniel Roy, an associate
professor of statistics at University of Toronto.
LinkedIn says an algorithm's integrity cannot be evaluated without
understanding its thinking.
It also maintains that tools like its CrystalCandle could help AI
users in other fields. Doctors could learn why AI predicts someone
is more at risk of a disease, or people could be told why AI
recommended they be denied a credit card.
The hope is that explanations reveal whether a system aligns with
concepts and values one wants to promote, said Been Kim, an AI
researcher at Google.
"I view interpretability as ultimately enabling a conversation
between machines and humans," she said. "If we truly want to enable
human-machine collaboration, we need that."
(Reporting by Paresh Dave; Editing by Kenneth Li and Lisa Shumaker)
[© 2022 Thomson Reuters. All rights
reserved.] This material may not be published,
broadcast, rewritten or redistributed.
Thompson Reuters is solely responsible for this content.
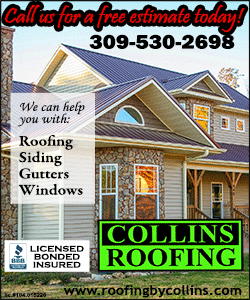 |